Key points
- While 'green-on-brown' weed detection is relatively simple, correctly identifying broadleaf and grass weeds within crops is more challenging
- University of Sydney researchers are using artificial intelligence and machine learning to develop a mechanical robot for 'green-on-green' in-crop weed control
With the ability to detect 'green-on-green' weeds, this non-chemical weed-control robot (pictured) may just be the answer to tackling herbicide resistance.
Site-specific weed management is an emerging tool in integrated weed management and precision agriculture involving detection of individual weeds and targeted control - either herbicide or physical.
The battle to beat herbicide resistance will likely require mechanical solutions, but broadscale cultivation is not compatible with minimum or no-till systems.
While a mechanical site-specific weed chipper has been developed to identify weeds as 'green-on-brown' using infrared reflectance, these systems are limited to fallow because they cannot tell the difference between a crop and a weed.
Green-on-green
Now, GRDC investment has enabled the Australian Centre for Field Robotics (ACFR), at the University of Sydney, to build and test a robotic solution for in-crop weed control.
The robot targets low-density weed populations shortly after early season weed spraying to reduce their contribution to the seedbank.
ACFR's prototype is designed to operate in the inter-row spacing between crop rows and has a wedge-shaped front and toe to push mature grain leaves aside.
The enclosed imaging canopy controls the lighting conditions to achieve consistent illumination and prevents mature grain leaves from cluttering the field of view.
Multispectral images captured by the system are analysed by an algorithm that classifies each pixel of the image into soil, weed or crop. Currently, any weeds identified are removed by a mechanical tool.
With enough data, deep learning methods can be trained to develop their own set of descriptive features without human intervention.
The real innovation in this research is the ability to perform 'green-on-green' detection - that is, detecting weeds among crop.
This problem is significantly more complex than 'green-on-brown' detection, as it requires the software to differentiate between the crop and various types of weeds.
This computational leap required deep learning, a field of artificial intelligence and machine learning that has proven to be scalable to difficult problems.
With enough data, deep learning methods can be trained to develop their own set of descriptive features without human intervention.
Field testing
Data was collected from a wheat crop at Narrabri, in New South Wales, to train the weed-detection algorithm.
When trained to locate broadleaf weeds in wheat, the algorithm was able to achieve a performance of 75 per cent - a result typical for this class of algorithm.
While broadleaf weeds have a distinctly different shape from wheat, getting the system to recognise grass weeds, such as ryegrass, is substantially more challenging.
In a crop with wheat, broadleaf weeds and ryegrass, the system scored 64 per cent.
Examples of human and machine interpretation of these images are shown in Figure 1.
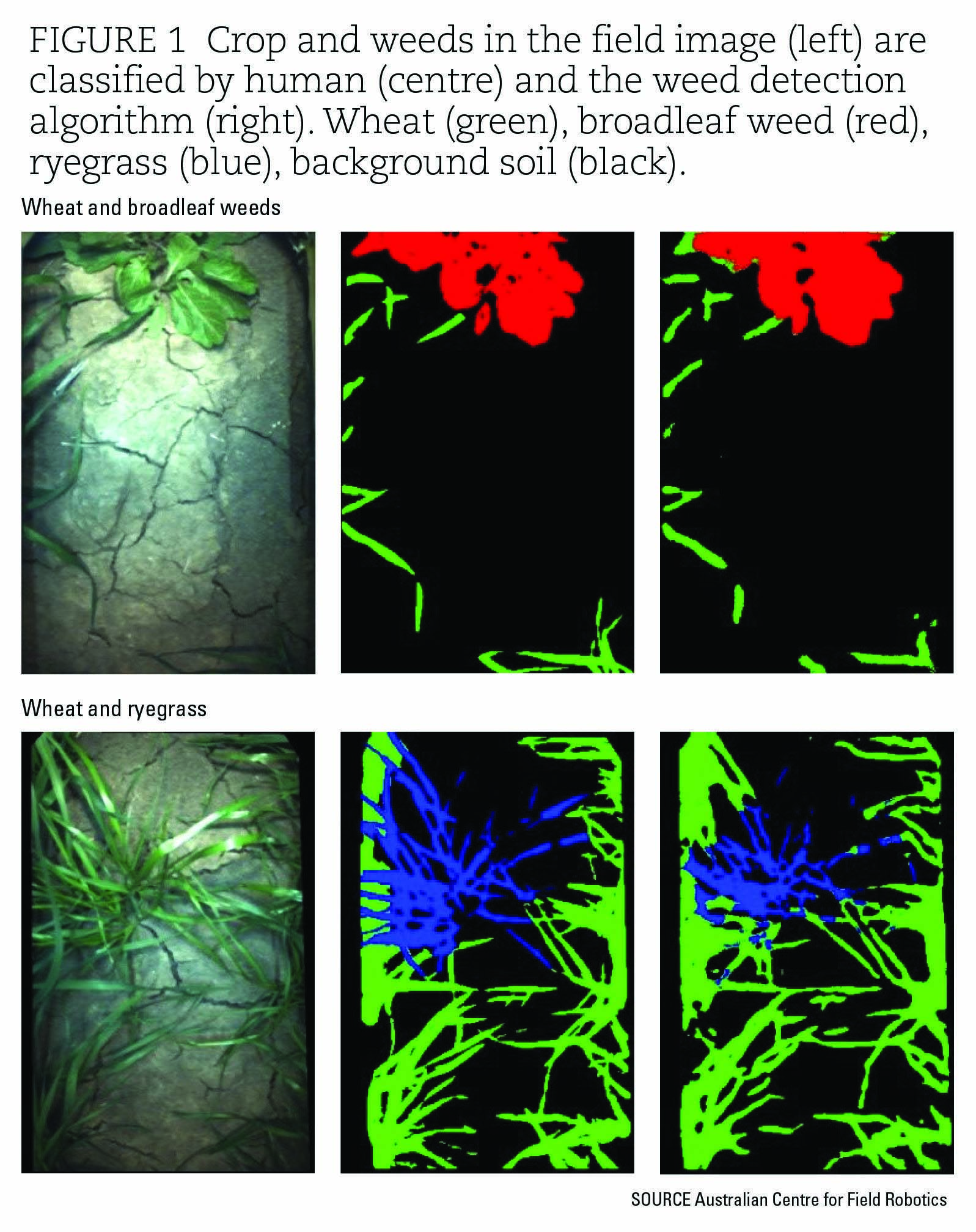
To demonstrate the concept of automated weed control, the system was run at the same farm where the data was collected.
The weeder was mounted to an autonomous vehicle designed at the ACFR for agricultural applications and fitted with a grinding unit to achieve non-chemical weed control (Figure 2).

FIGURE 2 The non-chemical, site-specific weeding device is designed to operate in the inter-row spacing between grain crop rows. The drawing has the left panel removed to show the internal imaging system and weeding grinder. Source: Australian Centre for Field Robotics.
During the test run, the weeder was able to reliably detect broadleaf weeds and deploy the grinder. It was not able to deploy the grinder on ryegrass with the same degree of reliability; however, it produced few false detections.
This research demonstrates that it is possible to reliably detect broadleaf weeds within a grain crop, but detecting grass weeds is a more challenging task that was demonstrated with moderate success.
Future research will focus on methods to better distinguish between grain crops and grass weeds and the potential to improve operation speeds.
Other non-chemical methods, such as laser and steam treatment methods, will also be investigated.
GRDC Research Code UOS1806-002AWX
More information: Dr Asher Bender, 02 9351 8173, a.bender@acfr.usyd.edu.au; Professor Salah Sukkarieh, 02 9351 8154, salah.sukkarieh@sydney.edu.au
NOTE: The authors would like to thank Javier Martinez, David Spray, Matthew Imhoff, Guy Coleman, Khalid Rafique and Professor Michael Walsh.